ADVERTISEMENT
Artificial Intelligence in Dermatology: Scribing and Documentation
© 2024 HMP Global. All Rights Reserved.
Any views and opinions expressed are those of the author(s) and/or participants and do not necessarily reflect the views, policy, or position of The Dermatologist or HMP Global, their employees, and affiliates.
Machine learning (ML) has unlocked a new generation of tools for outpatient health care. As discussed in my last article on this topic (see March edition), the utility of artificial intelligence (AI) for dermatologists is strictly dependent on the application of the technology. While lesion identification platforms off er minimal practical value, software focused on administrative tasks may greatly enhance our daily practice and workflow. Thousands of software as a service, or SaaS, companies are clamoring to sell products to the health care industry based on these algorithms using trendy ads that emphasize the power of AI. But which of these applications will make dermatologists more efficient? And—even harder to answer—which products make sense from a cost-benefit perspective?
Real-Time Ambient Assistants
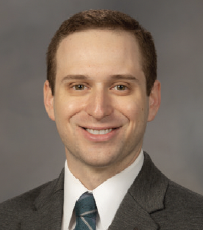
surgeon at Medical Dermatology Associates of Chicago. Find him on LinkedIn: https://www.linkedin.com/in/
ross-lane-pearlman-298942220
Documentation in the era of electronic health records (EHRs) is one of the most common causes of burnout among dermatologists.1 EHRs are mandatory and ubiquitous in 2024. They are also a double-edged sword when it comes to workflow: On one hand, documentation requirements and volume are more onerous than ever; on the other hand, it has never been easier to document sufficiently, at least for billing purposes. According to a recent study conducted by the Cambridge Health Alliance, clinical documentation consumes an average of almost 2 hours per day for outpatient physicians.2
A natural first question is how are AI-enabled clinical documentation technologies different from the voice-to-text technology that has been widely adopted over the past 2 decades? AI scribing refers to ML-enabled platforms that transcribe audio data from office visits and then analyze the data in probabilistic frameworks. The output should be an organized and concise note. Previously, natural language processing’s (NLP) integration into health care faced constraints due to the extensive manual annotation required for training datasets in a supervised learning framework. However, this changed dramatically with the advent of large language models, an advanced subset of NLP that employs self-supervised learning algorithms. These models are at the core of generative AI technologies, including OpenAI’s generative pre-trained transformer (ChatGPT) and Google’s Gemini (previously Bard).
The difference is in the processing of the incoming data. Standard voice-to-text technology simply transcribes audio into written language and is often utilized in dictated note preparation after a patient visit. On the other hand, most AI-scribing platforms are intended as ambient assistants and are used in real-time. AI scribes transcribe and subsequently analyze, edit, and summarize the patient encounter into a structured note. A microphone on an application-enabled smartphone, tablet, or computer listens to the patient encounter to collect data. Reputable platforms never store or record audio.
Although there is variation from platform to platform, most AI scribing products focus on summarizing and highlighting relevant conversation. However, just like working with a scribe, there is a learning curve associated with these tools, and the workflow depends on the type of AI scribing tool. There are 2 general categories of AI scribing software: pre-configured (aka standardized or fixed functionality) and trainable (aka adaptive). Standardized tools off er plug-and-play solutions where the NLP algorithms have already been tuned for introduction into a specialty setting. This type of standardization is likely to be encountered in large health care systems adopting AI scribing tools. A certain amount of editing and curation by the physician after generation of the note should be expected. Trainable tools come with a degree of out-of-the-box tuning but are designed to learn the physician’s preferences over time. Some may require direct clinician input to discern relevant data, structure notes to individual preferences, and improve scribing performance. These algorithms accommodate your personalized style, unlock high-resolution customizability, and decrease the need for editing and curation over time in exchange for upfront investment of effort.
In Practice
There have been several large-scale implementations of AI scribing platforms that can provide a glimpse into their efficacy. A large experimental launch of a standardized AI scribing tool was implemented in 2023 by Kaiser Permanente in California. They found that the average time spent in notes by primary care physicians adopting AI scribing decreased from 5.3 to 4.8 minutes. Stratifying for high, medium, and low use levels revealed that higher use was associated with the greatest time savings. This result likely reflects enhanced understanding by the clinician of the tool’s habits and more efficient editing and correction.3
Is this technology mature enough to replace the scribes that many of us have come to greatly depend on? It goes without saying that such significant shifts in clinical workflow must always be evaluated on a practice-to-practice basis. But luckily the appetite for adoption of new technology by all dermatologists in a practice need not be equivalent. Dr Joshua Ortego practices in Ocean Springs, MS, and has been using an AI scribing platform that integrates with his EHR for the past year. In our discussion, he reported great success with implementing AI scribing in his practice and integrating with a popular EHR. Before adopting the new platform, his nurses were functioning as his scribes. “Since implementing the AI scribe, my nurses’ lives have been made much easier. Now, all they have to do is start the AI before we walk in a room, and they are no longer tied to the computer. Also, the quality, detail, and accuracy of my notes has greatly improved.”
Conclusion
A plethora of AI scribing options are now on the market that allow for implementation by single physicians. Axios Pro recently conducted a multi-product review of AI medical scribing platforms, evaluating both pricing and accuracy. Platform costs ranged from around $120 per user per month to $600.4 Before choosing a platform for your practice, ensure that dermatology specific support has already been developed for the product. It is also important to explore the quality of the integration with your EHR. Ask how many clients use the same EHR with the software and consider talking with others who have adopted the AI scribing software in the same EHR environment about their experience.
References
1. Colon A, Gillihan R, Motaparthi K. Factors contributing to burnout in dermatologists.
Clin Dermatol. 2020;38(3):321-327. doi:10.1016/j.clindermatol.2020.02.002
2. Gaff ney A, Woolhandler S, Cai C, et al. Medical documentation burden
among US offi ce-based physicians in 2019: a national study. JAMA Intern Med.
2022;182(5):564-566. doi:10.1001/jamainternmed.2022.0372
3. Tierney AA, Gayre G, Hoberman B, et al. Ambient artifi cial intelligence scribes
to alleviate the burden of clinical documentation. NEJM Catal Innov Care Deliv.
2024;5(3). doi:10.1056/CAT.23.0404
4. Brodwin E. AI medical scribes vary widely by price and other features. Axios
Pro Online. March 21, 2024. Accessed June 21, 2024. https://www.axios.com/pro/
health-tech-deals/2024/03/21/ai-medical-scribes-comparison-price-accuracyabridge-
suki-ambience-nuance